This week Anbu Valluvan Devadasan et all published a paper titled “E-SMOTE: Entropy Based Minority Oversampling for Heart Failure and AIDS Clinical Trails Analysis.”
I reached out to Anbu to chat about the Entropy-based Synthetic Minority Oversampling Technique (SMOTE) which uses a subset of the dataset from the minority class to address overbias of a majority class. Anbu explained that the team used entropy as a metric to assess influence of a minority class on outcomes and outperform conventional SMOTE oversampling techniques in some cases.
Results illustrated that both SMOTE and E-SMOTE techniques should be considered for addressing imbalanced data samples as they can be used to address class imbalance.
Anbu is excited and looking forward to dig deeper into which data characteristics affect metrics and how.
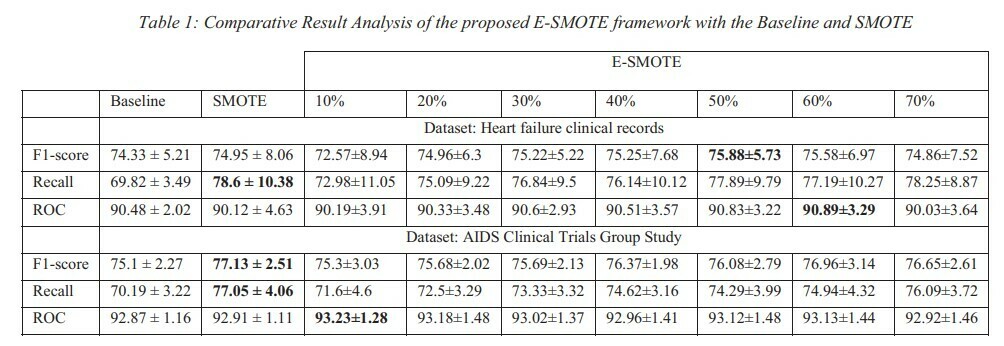
Anbu’s open to chat about his work. If you’re interested, ping him - linkedin.com/in/anbuvalluvan.
S. Veerla, A. V. Devadasan, M. Masum, M. Chowdhury and H. Shahriar, "E-SMOTE: Entropy Based Minority Oversampling for Heart Failure and AIDS Clinical Trails Analysis," 2024 IEEE 48th Annual Computers, Software, and Applications Conference (COMPSAC), Osaka, Japan, 2024, pp. 1841-1846, doi: 10.1109/COMPSAC61105.2024.00291.
keywords: {Measurement;Training;Uncertainty;Clinical trials;Market research;Entropy;Stability analysis;Imbalanced class;Entropy;Sampling;Machine Learning;SMOTE}