Google researchers have unveiled GameNGen, a groundbreaking game engine entirely driven by AI that can simulate intricate video games in real-time. This innovative technology was showcased by recreating the iconic first-person shooter, Doom.
GameNGen employs a neural network to generate game frames at more than 20 frames per second, creating visuals that closely mirror the original game. The AI model underwent a two-phase training process: initially, an AI agent was taught to play Doom, followed by training a separate model to predict the next frame based on previous actions and frames.
“We introduce GameNGen, the first game engine entirely powered by a neural network, capable of real-time interaction within a complex environment over extended periods and at high quality,” the researchers detailed in their paper.
This technology holds the potential to revolutionize game development by enabling the creation and modification of games through text descriptions or example images, moving away from conventional coding methods. This could make game development more accessible and cost-effective.
Despite its advancements, GameNGen has some limitations, including the AI’s restricted memory of past events and discrepancies between the AI's gameplay and that of human players.
The team envisions that their work will pioneer a new era in interactive software systems, with implications extending beyond video games to various other applications.
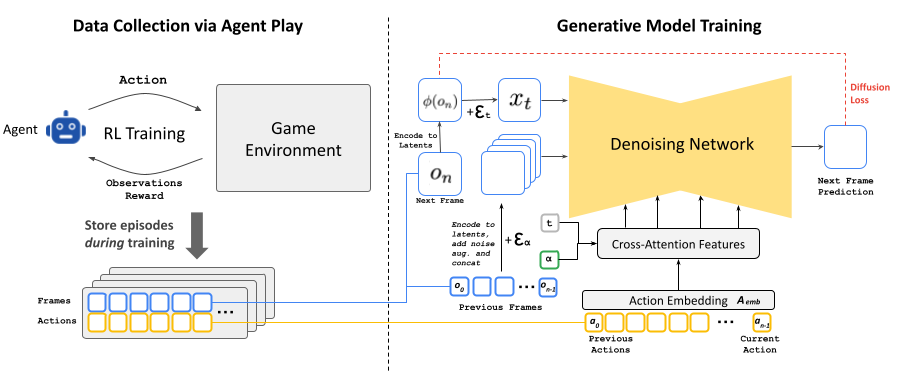
Abstract
We present GameNGen, the first game engine powered entirely by a neural model that enables real-time interaction with a complex environment over long trajectories at high quality. GameNGen can interactively simulate the classic game DOOM at over 20 frames per second on a single TPU. Next frame prediction achieves a PSNR of 29.4, comparable to lossy JPEG compression. Human raters are only slightly better than random chance at distinguishing short clips of the game from clips of the simulation. GameNGen is trained in two phases: (1) an RL-agent learns to play the game and the training sessions are recorded, and (2) a diffusion model is trained to produce the next frame, conditioned on the sequence of past frames and actions. Conditioning augmentations enable stable auto-regressive generation over long trajectories.
Data Collection via Agent Play: Since we cannot collect human gameplay at scale, as a first stage we train an automatic RL-agent to play the game, persisting it's training episodes of actions and observations, which become the training data for our generative model.
Training the Generative Diffusion Model: We re-purpose a small diffusion model, Stable Diffusion v1.4, and condition it on a sequence of previous actions and observations (frames). To mitigate auto-regressive drift during inference, we corrupt context frames by adding Gaussian noise to encoded frames during training. This allows the network to correct information sampled in previous frames, and we found it to be critical for preserving visual stability over long time periods.
Latent Decoder Fine-Tuning: The pre-trained auto-encoder of Stable Diffusion v1.4, which compresses 8x8 pixel patches into 4 latent channels, results in meaningful artifacts when predicting game frames, which affect small details and particularly the bottom bar HUD. To leverage the pre-trained knowledge while improving image quality, we train just the decoder of the latent auto-encoder using an MSE loss computed against the target frame pixels.